10:00 - 11:45
| | IEEE DLP Talks
|
10:00 - 10:15
| | Opening Ceremony
|
10:15 - 11:00
| | Jacek M. Zurada: Towards Better Understanding of Data: Constrained Learning of Latent Features in Neural Networks
|
| | Presentation is available: 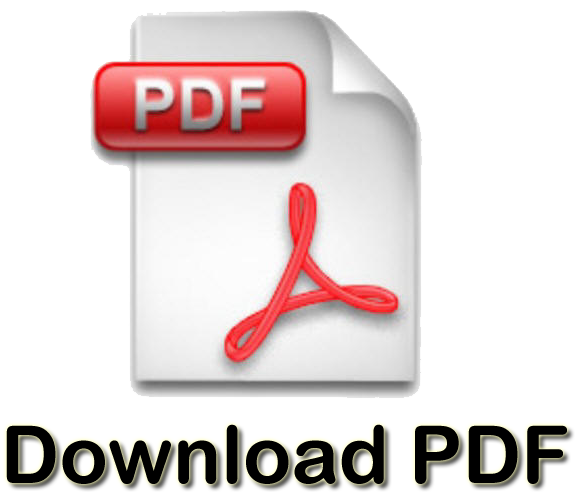
|
| | Abstract: Learning models that build hierarchies of concepts are inherently difficult to interpret and understand. Hence, convoluted mappings and cancellations of terms performed within neural networks with one hidden layer or more make them less than transparent. However, learning with meaningful constraints either within classic or recently proposed architectures allows for better extraction of discriminative features. The discriminative features are understood here as parts of original sets of objects. Further, they are useful only when they can be superimposed and reconstructed with as low a reconstruction error as possible.
Three techniques are discussed that meet the criteria outlined above. (1) They use supervised and unsupervised learning. Nonnegative Matrix Factorization is one of the efficient techniques that reduces the number of basis functions and allows for extraction of latent features that are additive and hence interpretable for humans. (2) A classic error-back propagation architectures can also be trained under the constraints of non-negativity and sparseness. The resulting classifiers allow for identification of parts of the objects encoded as receptive fields developed by weights of hidden neurons. The results are illustrated with MNIST handwritten digits classifiers and Reuters-21578 text categorization. (3) A constrained learning of sparse encoding representation using non-negative weights of an auto-encoder also allows for discovery of additive latent factors. Our experiments with MNIST, ORL face and NORB object datasets compare the auto-encoding accuracy for various training conditions. They indicate an enhanced interpretability and insights through identified parts of complex input objects traded-off for a small reduction of recognition accuracy or classification error. Although for the sake of interpretability these models discuss only shallow networks, their training strategies parallel those used in multi-layer deep learning.
|
11:00 - 11:45
| | William A. Gruver : Algorithmic Trading Systems
|
| | Presentation is available: 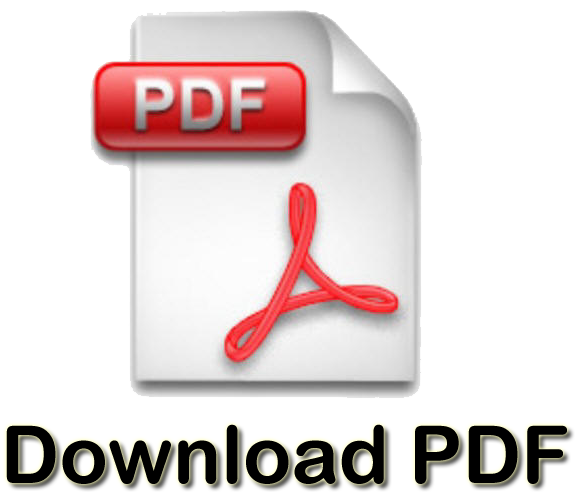
|
| | Abstract
: Although many of the world’s markets have rebounded since the crash of 2008, many believe a major correction is overdue. Some even claim that the markets are rigged in favor of those who employ high speed network connections to the exchanges in order to front run traders. Nevertheless, it is acknowledged that the change from open outcry pits to fully electronic exchanges, and the increased use of high frequency trading, requires a new approach to investment decisions.
The purpose of this plenary is to introduce practical aspects of data analysis and trading of the financial markets. Topics to be discussed include market moving events, market psychology, high frequency trading and dark pools, time frames, supply/demand levels, technical indicators, and strategies for swing trading.
Examples will be presented from the live markets to illustrate the techniques being presented. Although the examples will be based on data from U.S. equity and commodity exchanges, the methodology is applicable to the analysis and trading of markets worldwide.
The presentation will conclude with future opportunities for research in the development of algorithmic trading systems.
|